ASD | Soil salinity inversion based on differentiated fusion of satellite image and ground spectra
Soil salinization is an important limiting factor in agricultural development, ecological security, and sustainable land use. Accurately and rapidly obtaining information related to regional soil salinization and its geographical distribution is a precondition for the rational control and utilization of salinized soil. The traditional method of soil salinity mapping is time consuming and laborious. Ground-based methods, such as those based on electromagnetic induction meters, may not meet the temporal needs of regional monitoring. Due to the wide availability of remote sensing data from different sensors and platforms, soil salinity inversion based on the fusion of multisource remote sensing data is becoming a reality. However, existing fusion methods mainly use the average relationship from samples, which does not consider the differences in the relationships among samples.
Based on this, to fill the research gap, in this article, a group of scientists from Shandong Agricultural University and University of Wisconsin-Madison conducted field surveys in the Kenli District (37°24′-38°10′ N, 118°15′-119°19′ E) of Shandong Province, which encompasses the mouth of the Yellow River in China from October 4-8, 2018.
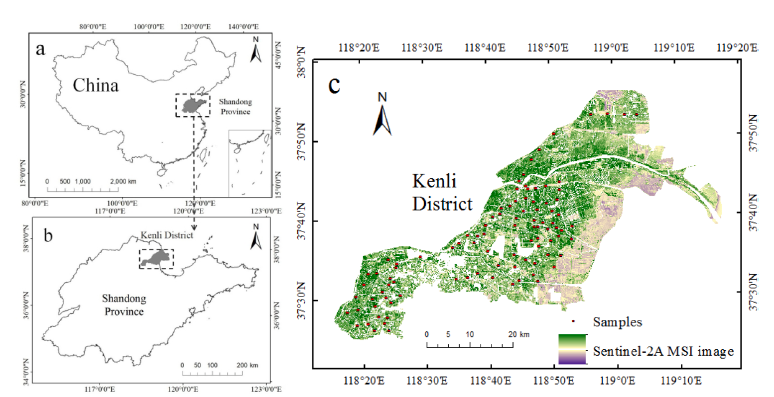
Location of the study area: (a) China; (b) Shandong Province; and (c) Sentinel-2A MSI image of the study area with the sample locations
Soil samples were naturally air dried, crushed, and evenly mixed. The extracted solution was prepared at a 1:5 soil–water ratio, and the total content of soil salinity was determined. And the spectral signatures of airdried soil samples were measured indoors with an ASD FieldSpec 4 instrument having a wavelength range of 350–2500 nm. Maintaining similar dates for field sampling and satellite images is a prerequisite for the fusion of satellite and ground spectra. The Sentinel-2A MSI image whose ID is 50SPG was acquired on October 4th, 2018, and it was provided by the European Space Agency (ESA) as an atmospheric apparent reflectance product with accurate geometric corrections. The Sentinel-2A MSI image has 13 spectral bands, from visible to shortwave-infrared bands, and three spatial resolutions: 10 m, 20 m, and 60 m.
A differentiated fusion method for determining satellite and ground spectral variables of soil salinity according to the differences among samples is proposed to increase the regional inversion precision. Nonnegative matrix factorization was employed to decompose soil salinity spectral variables from the Sentinel-2A Multi-Spectral Instrument (MSI) image and the simulated spectra on ground spectra. Then, the base spectra matrix of soil salinity was from the simulated data, and the weight coefficient matrix was obtained from the Sentinel-2A MSI data as the differentiated correction coefficients. By multiplying the base matrix and weight matrix, the spectral variables were reconstructed.
【Results】
The shapes of the ground spectra curve and the simulated spectra curve are consistent, which suggests that the simulated spectra provide a good approximation of the ground spectra. A comparison of the simulated spectra and the Sentinel-2A MSI image surface reflectance values indicates that the overall trends of the two curves are similar; notably, the bands near the green wavelength and the areas beyond the NIR band are highly similar.
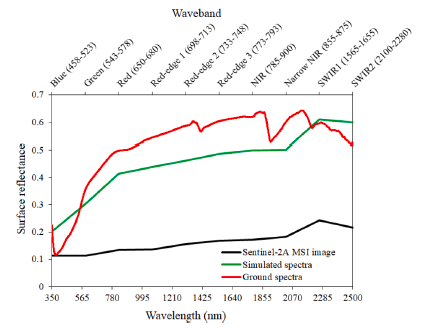
Comparison of ground, simulated, and Sentinel-2A MSI image surface reflectance values.
The correlation coefficients between the MSISVs and the soil salinity ranged from 0.70 to 0.74, and those between the SSVs and the soil salinity ranged from 0.75 to 0.79. The correlations between the SSVs and the soil salinity were better than those between the MSISVs and the soil salinity. This result should have occurred mainly because the ground spectra were measured indoors without the influence of other factors, such as soil moisture and measuring conditions.
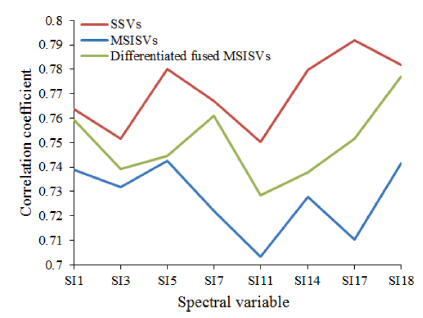
Correlation between spectral variables and soil salinity.
According to the definitions and thresholds of the evaluation indicators, the results indicate that the soil salinity of the study area can be predicted using this model.
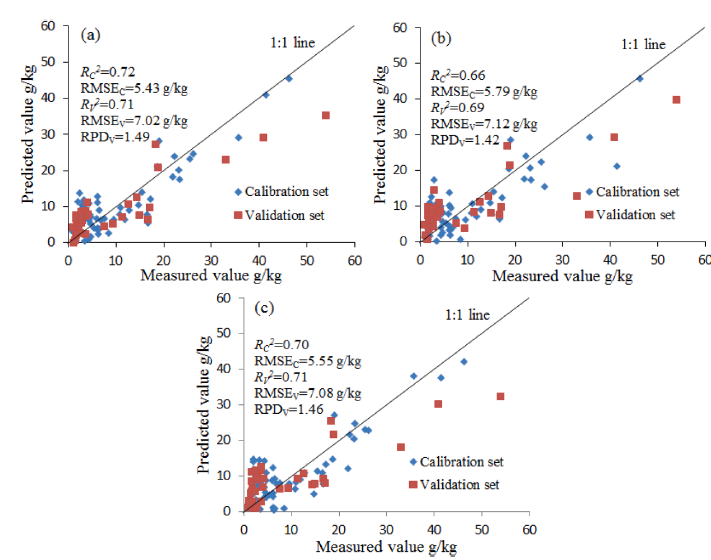
Scatter diagrams of (a) the differentiated fused model with the cjlin algorithm; (b) the average-ratio adjusted model and (c) the differentiated fused model with the als algorithm.
The following figure shows that most of the sample points are distributed in the corresponding grade of salinized soil, and only a few points deviate.
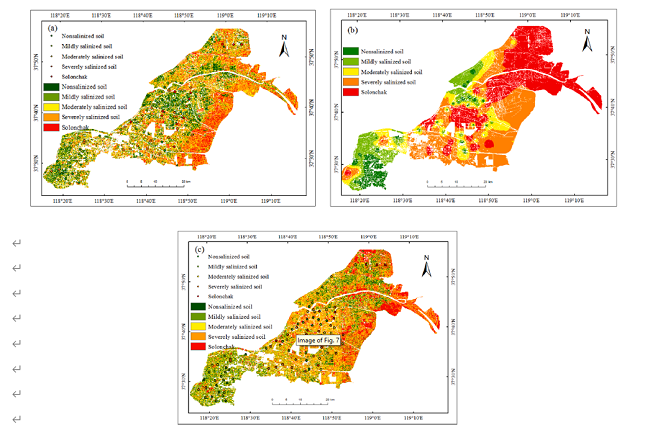
(a) Distribution map on the differentiated fused model; (b) interpolation map and (c) distribution map on the average-ratio adjusted model of soil salinity in the Kenli District.
Based on analyses of the salinized soil in the Yellow River Delta, this paper proposes a differentiated fusion method of ground spectra and satellite image data using NMF for the inversion of soil salinity. The results show that this fusion method strengthens the correlation between Sentinel-2A MSI image reflectance values and soil salinity and improves the precision of regional soil salinity inversion based on satellite images. This research not only can promote the high-precision, large-area, and rapid acquisition of regional soil salinity, but also is significant for understanding and controlling salinized soil and for sustainable agricultural development in the study area. Moreover, this method can enrich the technology and approach of multisource remote sensing data fusion. For regional soil quantitative inversion, selecting a method for the fusion of multisource remote sensing data according to the differences in regional soil properties is recommended.
6375822300910717374488093.pdf