Application of Resonon Pika L Hyperspectral Imaging on the estimation of amino acid contents in maize leaves
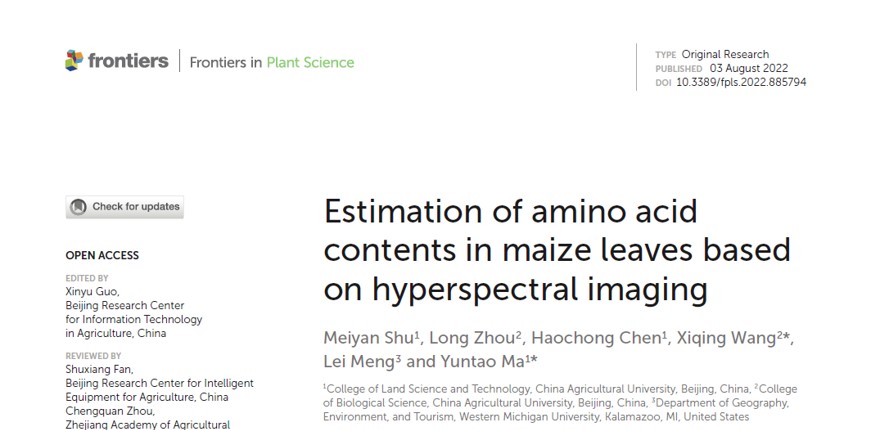
Maize is one of the most important crops in the world. In maize growth, nitrogen (N) is one of the most important nutrient elements. The nitrogen translocation in maize leaves was mainly in the form of glutamine. The maize yield is correlated well with the amino acids in leaves, such as glutamine, glutamate, alanine, aspartate, and asparagine at the grain filling stage. Therefore, accurate, and rapid estimation of amino acid contents in maize leaves is of great significance in improving maize yield estimation and nitrogen use efficiency. The spectrophotometry, chemical analysis, and mass spectrometry are the main methods for determining the amino acid content. These methods can estimate a variety of amino acids and have the advantages of high sensitivity and accuracy. However, all of them need to damage samples and require complex sample processing, low throughput, and high price. The hyperspectral imaging technology provides a new method for estimating physiological and biochemical parameters of crops with the advantages of being rapid, high throughput, and non-destructive, and it has been used for high-throughput screening of crop phenotypic traits. However, the research on the application of hyperspectral data in estimating the amino acid contents in fresh maize leaves is very limited.
Based on this, in the attached article, a group of scientists from China Agricultural University took maize leaves as the research object, and explored the feasibility of estimating various amino acid contents in fresh maize leaves using hyperspectral imaging data. They conducted two independent experiments with variable N fertilizer applications. A hyperspectral image acquisition system (Resonon Pika L Hyperspectral Imaging) was used to collect the hyperspectral images of maize leaves, and 24 amino acid contents were determined. First, the sensitive band range and sensitive bands of each amino acid were selected by the coefficient of variation (CV) and partial least squares regression (PLSR) coefficient tests. Then, the models of 24 amino acid contents were established based on the reflectance of all bands, sensitive band range, and sensitive bands, respectively. Finally, the samples that were not involved in model construction were used to verify the model accuracy of each amino acid.
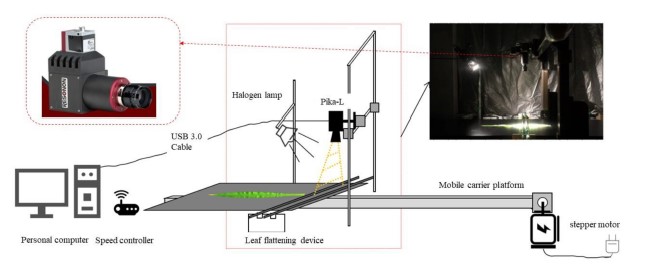
Hyperspectral images acquisition system.
Results:
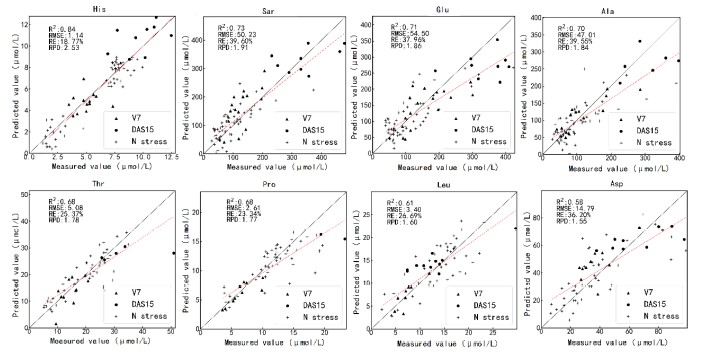
Scatterplot of the measured value against the predicted value of the various amino acid contents by the optimal estimation method using test set.
Conclusions:
The regression coefficient test of PLSR found that the sensitive bands of most amino acids were in the ranges of 505.39-604.95 nm and 651.21-714.10 nm. The model estimations of the 24 amino acid contents were constructed and validated based on the reflectance of all bands, sensitive band range, and sensitive bands. The authors selected the optimal estimation method for each amino acid. The estimation accuracy of the content of b-aminobutyric acid, ornithine, citrulline, methionine, and histidine was better than other amino acids, with R2, RE, and RPD of the test set in the range of 0.84–0.96, 8.79%–19.77%, and 2.58–5.18. The estimation accuracies of the content of sarcosine, alanine, glutamic acid, proline, threonine, leucine, and aspartic acid were normal, with R2, RE, and RPD of the test set in the range of 0.58–0.73, 23.23%– 39.69%, and 1.56–1.94. The performance of the other amino acid models was relatively poor. This study can provide a reference for monitoring the traits of breeding materials based on hyperspectral technology.