Application of Resonon Pika L Hyperspectral Imaging on Individual tree segmentation and tree species classification in subtropical broadleaf forests
Accurate information on tree species is essential in sustainable forest management, ecosystem service assessment, biodiversity monitoring, and ecological environment protection. Therefore, efficient, and cost-effective ways for classifying individual tree species are highly desirable. Traditional ground survey of tree species is time-consuming, labor-intensive, and expensive, which is hard to implement in large area. Remote sensing can acquire feature information over large areas with low cost. Many remote sensing data, such as ultrahigh-resolution RGB, airborne multispectral, hyperspectral, and light detection and ranging (LiDAR) data, have been used in individual tree segmentation and tree species classification. However, many of the previous studies were conducted using single or two types of data. Few studies have been conducted to integrating these three kinds of remote sensing data for tree species classification.
Based on this, in the attached article, a group of scientists presented a new approach for individual tree species classification in subtropical broadleaf forests using UAV LiDAR, hyperspectral (Resonon Pika L Hyperspectral Imaging), ultrahigh-resolution RGB data and field data. They first developed a new watershed-spectral-textural-controlled normalized cut (WST-Ncut) algorithm for individual tree segmentation, then conducted the classification by integrating UAV LiDAR, hyperspectral, and ultrahigh-resolution RGB data, from which the structural, spectral, and textural features of individual trees were extracted, respectively. And finally, the overall accuracy (OA) and kappa coefficient (k) were used for accuracy evaluation. The specific objectives of this study are: 1) to assess the accuracy of individual tree segmentation of the proposed WST-Ncut algorithm in subtropical broadleaf forests; 2) to evaluate the effectiveness and improvement in subtropical broadleaf tree species classification using a fusion of UAV LiDAR, hyperspectral, and ultrahigh-resolution RGB datasets, in comparison with using these data alone; and 3) to explore the effect of the accuracy of individual tree segmentation and the number of tree species on the accuracy of tree species classification.
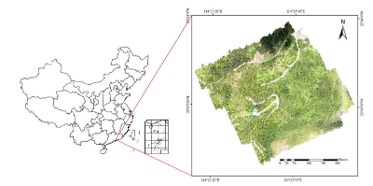
The location of the study area in Julongshan Park, Shenzhen City of southern China.
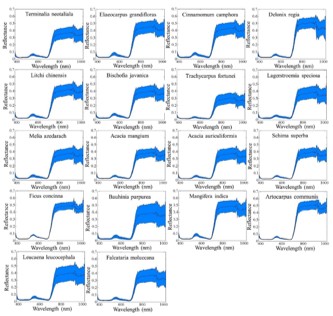
The mean and ± standard deviation of the reflectance of 18 tree species in the field at 383–1020 nm wavelengths.

The mean spectral reflectance of 18 tree species in the field at 383–1020 nm wavelengths.
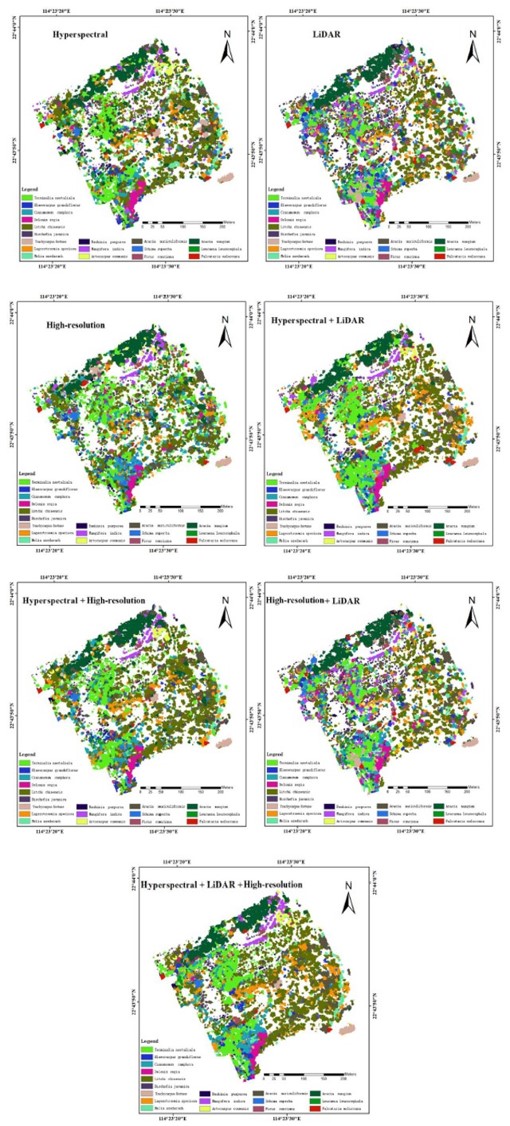
The tree species distribution maps derived from the seven kinds of feature combinations.
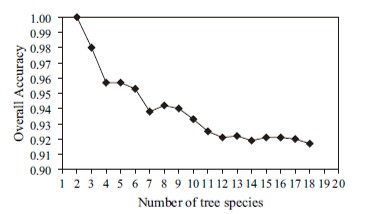
The relationship between the overall classification accuracy obtained when using all features and the number of tree species.
Conclusions:
In this study, the authors used UAV LiDAR, hyperspectral, and ultrahigh resolution RGB data to classify 18 tree species in subtropical broadleaf forests at tree level. They proposed a watershed-spectral-textural controlled normalized cut (WST-Ncut) algorithm to delineate individual trees at first. Results showed that the WST-Ncut algorithm was suitable for delineating individual trees in subtropical broadleaf forests (Recall = 0.95, Precision = 0.86, and F-score = 0.90), which could reduce the over-segmentation. LiDAR-derived vertical structural features, hyperspectral-derived spectral features, and ultrahigh-resolution RGB data-derived textural features were complementary in tree species classification. The classification results showed that combining the three datasets could effectively discriminate the 18 tree species, obtaining the best classification accuracy (Overall accuracy = 91.8%, and Kappa = 0.910), which was 10.2%, 13.6%, and 19.0% larger than that of using spectral features, structural features, and textural features alone, respectively. In addition, results showed that better individual tree segmentation would lead to higher accuracy of tree species classification, and the increase of the number of tree species would result in the decline of classification accuracy.